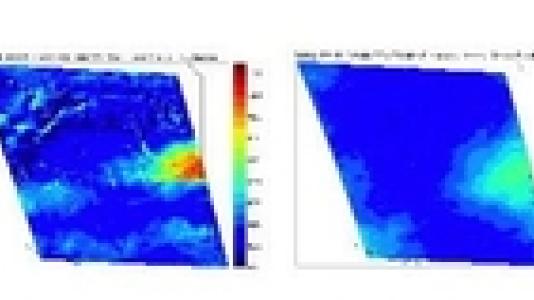
Solar power is becoming increasingly important for energy applications. Such solar-enabled applications depend on solar irradiation – the amount of power per unit area that reaches the Earth’s surface. And as people in climates such as the Midwest well know, the amount of sunlight that reaches the Earth varies significantly during the year, day, hour, and minute by minute. Yet calculating solar irradiance accurately is critical for any solar-power conversion operation.
The most widely used methods for solar irradiation forecasting rely principally on local data. But since weather fluctuations exhibit a nonlocal behavior, the accuracy of such forecasts degrades rapidly as the forecasting window increases. Longer forecasts have been developed, for example, that extract cloud structure information from satellite images. Like the other techniques, however, these sky image-based techniques are difficult to use and sometimes unable to quantify the uncertainty in their predictions.
“Yet it is exactly this uncertainty that helps quantify the potential operational risks and allows stakeholders to properly price them,” said Emil Constantinescu, a computational mathematician at the U.S. Department of Energy’s (DOE) Argonne National Laboratory.
To address this issue, Constantinescu, in collaboration with Mihai Anitescu (a senior computational mathematician at Argonne) and Ilias Bilionis (a former postdoctoral researcher at Argonne and now an assistant professor at Purdue University), developed a new model that can capture uncertainties and make predictions in real time with minimal computational resources. The model uses solar irradiation data from satellite scans of the continental United States. To remove the effect of the Sun’s movement on the observed field, the researchers divide it with a clear sky model, which approximates the solar irradiation when no clouds are present. The result is a clear sky index field.
One of the aims of the research is to learn the dynamics of this field. To deal with its high dimensionality – involving 160,000 (400x400) dimensions – the team devised a dimensionality reduction technique (see Fig. 1). They then modeled the dynamics of the reduced representation as a dynamical system. The dynamics are represented in a nonlinear way by a recursive Gaussian process.
“Such processes have been used extensively in human motion modeling,” acknowledged Anitescu. “But a key feature of our approach is the use of Bayesian principles. Thanks to these, we are able not only to make best estimates but also to produce probabilistic forecasts.”
The process involves characterizing in a probabilistic manner all the information about the dynamics that can be obtained from the observations. Iterative sample paths of the irradiation field can then be taken that go arbitrarily into the future.
To test the usefulness of the model, the researchers compared their model results with those using only ground data, the prevailing forecast method. In an 8-hour-ahead forecast, the ground model did better initially, but its performance deteriorated gradually. Indeed, for longer predictions, the new model produced significantly better predictions and uncertainty estimates for the solar radiation than did models with only site data. The uncertainty estimates for the ground model are reveal that those predictions are completely unreliable.
The experiments were based on data from Lemont, OK. Anitescu noted, however, that since data is available over the entire United States, the new model can be used to produce forecasts at any site in the United States with comparable accuracy. “This may prove important for very distributed solar energy systems,” he said.
For further information, see the full paper:
I. Bilionis, E. M. Constantinescu, and M. Anitescu, “Data-Driven Model for Solar Irradiation Based on Satellite Observations,” Solar Energy 119: 22-38, 2014.